Understanding the Landscape of AI Rankings
Written by Eric Verbrugge
Lost in AI rankings? This guide charts the landscape—from model benchmarks to national readiness and compute lists—explaining how granularity and update cadence shape what each ranking reveals.
Artificial intelligence is evolving at incredible speed, and a diverse set of stakeholders—policymakers, AI tool providers, researchers, journalists, and professionals—need reliable dashboards to keep track.
AI rankings serve as that dashboard, yet their value depends fundamentally on granularity—the level of detail each ranking captures. Some zoom in to evaluate individual AI-models on narrow technical benchmarks. Others zoom out to rank tools and platforms by real-world adoption or assess the companies behind them for safety and ethics. Broader are indices that compare entire cities, regions, or countries on policy readiness, talent or infrastructure.
Granularity also shapes update cadence. Fine-grained AI-model leaderboards refresh in near-real time; tool popularity charts update weekly or monthly; country-level policy indices often appear annually. Understanding these layers and rhythms lets you choose the right ranking for the question at hand.
To make sense of this complex landscape, we’ve organized the most important rankings into seven core categories:
- AI Model Performance
- Adoption & Popularity of AI
- Responsible & Ethical AI Rankings
- AI Governance & Policy Readiness
- Infrastructure & Computing Power
- Talent & Education
- Sector‑Specific AI Rankings
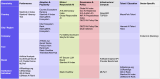
AI Performance
Performance rankings measure how well AI systems—particularly large language models (LLMs)—understand, reason, and respond. While many evaluations target individual models, newer indices aggregate these metrics to compare universities, researchers, and national ecosystems. Some of the most important benchmarks in this field are:
- Artificial Analysis – Aggregates model scores across multiple intelligence dimensions to rank general‑purpose LLMs (3 hr)
- Chatbot Arena (UC Berkeley) – Head‑to‑head arena where users rank models anonymously in real‑time conversations (~30 min)
- CSRankings (AI Subfields) – Ranks universities and individual researchers by publications in top-tier AI conferences, highlighting institutional and personal research performance (quarterly)
- GLUE & SuperGLUE – Popular natural language processing benchmarks for evaluating general language understanding (daily)
- MLPerf (MLCommons) – Tracks training and inference performance across a range of AI tasks and hardware platforms (biannual)
- Stanford AI Vibrancy Tool – Compares countries on research output, citation impact and model performance to gauge national AI strength (annual)
Together, these rankings offer a clear view of which AI models—and, at broader scales, which countries and institutions—are leading on specific tests. Yet they still don’t tell the full story of how models perform in the real world, nor how popular or widely adopted they are among users.
Adoption & Popularity of AI
Performance tells us what AI can do; adoption shows what people actually use. On the tool level there are leaderboards such as AITools.xyz and our own RankmyAI, which track popularity signals like traffic and reviews. There are also fully crowd‑voted boards—most notably GenAI Works and ThereIsAnAIForThat—that rely on up‑voting and can be easily manipulated, so they should be interpreted with caution. Other notable rankings include:
- AI Ecosystem Country / City Rankings (StartupBlink) – Ranks ecosystems on startup density, research output, and public‑private activity (annual)
- Stanford AI Index – Comprehensive yearbook of global AI metrics, from research to investment (annual)
Beyond individual tools and developer surveys, broader adoption trends can also be observed at the city and country level. Rankings like the StartupBlink AI Ecosystem Country Rankings and the Stanford AI Index offer a macro perspective on national AI momentum — measuring factors like startup density, research output, and public-private activity. At the city level, the StartupBlink AI Ecosystem City Rankings highlight growing urban hubs that are leading in AI development and ecosystem strength.
These rankings vary in granularity—from individual tools and frameworks to entire national ecosystems—and are particularly useful for spotting where real‑world traction is emerging.
Responsible & Ethical AI
As AI systems become more powerful and more embedded in daily life, concerns around fairness, accountability, and harm mitigation have taken center stage. Responsible‑AI rankings gauge how safely and ethically AI is being developed and deployed. These rankings are applied at multiple levels of granularitym— from countries and companies down to individual models. Some rely on expert review or qualitative assessment, while others use structured evaluation frameworks.
- AI & Democratic Values Index (CAIDP) – Grades national AI strategies on alignment with democratic principles such as accountability and non‑bias (annual)
- FLI AI Safety Scorecard – Rates major AI companies on internal governance, safety measures, and transparency (ad‑hoc)
- Global Index on Responsible AI (GIRAI) – Evaluates 130+ countries on inclusion, transparency, and rights‑based governance (annual)
- Hugging Face LLM Safety Leaderboard – Tests LLMs directly for safety, robustness, and toxicity (realtime)
- Storyful AI Index – Captures public perception and media narratives around AI ethics (monthly)
Responsible‑AI rankings don’t always use quantitative but also qualitative metrics; Still, they provide essential signals about trust, intent, and oversight—all of which shape how AI is perceived and governed.
Governance & Policy Readiness
These AI rankings assess how prepared governments and institutions are to guide the development and deployment of AI. The rankings focus on national strategies, legal frameworks, innovation ecosystems, and policy coordination. These rankings operate primarily at the country level, though some (like OECD’s observatory) offer regional or subnational data where available.
- AI Preparedness Index (IMF) – Assesses 170+ countries on digital infrastructure, governance, and human capital (annual)
- Global AI Index (Tortoise Media) – Combines investment, innovation, and implementation to rank 60+ countries (annual)
- Government AI Readiness Index (Oxford Insights) – Scores governments on strategy, infrastructure, skills, and data availability (annual)
- Latin American Artificial Intelligence Index (ILIA, CEPAL) – Benchmarks Latin‑American countries on strategy, capacity, and impact (annual)
- OECD AI Policy Observatory – Living database that tracks national and regional AI policy developments (continuous)
- Stanford Global AI Vibrancy Tool – Fine‑grained comparisons of research, policy, and institutional strength (ongoing)
Policy and governance rankings are often used by governments, international organizations, and regulators to benchmark progress — or to identify gaps in leadership, infrastructure, or accountability.
Infrastructure & Computing Power
Behind every advanced AI model lies enormous computational infrastructure. Rankings in this category assess which nations, institutions, or systems provide the muscle required to train and deploy large‑scale AI.
- Artificial Analysis API Provider Benchmark – Tracks how fast and affordable leading LLM providers are (3 hr)
- Green500 – Ranks supercomputers by energy efficiency (semi‑annual)
- MLPerf – See above; also benchmarks hardware systems for AI workloads (biannual)
- TOP500 – Lists the world’s most powerful supercomputers (semi‑annual)
These rankings typically apply at the country and institutional level, reflecting national capacity and hardware investment. Some also offer insight into specific hardware vendors or data‑centre strategies.
Infrastructure rankings don’t just measure performance—they reveal where AI capability can scale. Access to compute is often the hidden driver behind who gets to build state‑of‑the‑art systems.
Talent & Education
AI progress depends not just on models and machines, but on people. Rankings in this category help track where AI talent is trained, concentrated, and deployed. They reflect the academic foundations of the field as well as its geographic distribution.
- AIRankings.org – Evaluates institutions, researchers, and cities by AI publication metrics (monthly)
- CSRankings – Ranks computer‑science departments by top‑tier conference publications (quarterly)
- Harvard AI Talent Hubs – Identifies global metro areas attracting AI professionals (annual)
- MacroPolo AI Talent Tracker – Maps global movement of AI PhDs and top researchers (annual)
- QS AI Rankings – Rates universities on AI‑specific academic output and reputation (annual)
These rankings span multiple levels—from individual researchers to institutions, cities and countries. Together, they provide a picture of how knowledge flows through the AI ecosystem.
Sector‑Specific Rankings
While most AI rankings focus on models, tools, or countries, a few attempt to measure adoption within specific industries. These sector-specific rankings are still rare but valuable, as they show how AI is being applied in practice.
A clear example is the Evident AI Index, updated annually, which assesses the AI maturity of major banks, looking at transparency, innovation, leadership, and talent. For now, it's one of the few structured comparisons within a single sector.
What the Rankings Really Reveal
AI rankings help us interpret a landscape that’s changing fast. They offer structure, comparability, and a way to track progress—whether you’re evaluating national readiness, company ethics, model performance, or tool adoption.
But these rankings also vary widely in focus and depth. Some measure public perception, others measure scientific output. Some rank countries, others rank tools, models, or individual researchers. That’s why it’s essential to read them not as absolute scores but as signals—each one revealing a different dimension of the AI ecosystem.
At RankmyAI, we currently focus on tool and company‑level usage and adoption, but that is only our first analytical layer. Our platform already shows rankings of tools and companies on the city and country level. In the future, we aim to develop rankings of countries, regions and cities by adding up all tool scores within a specific granularity. These aggregate scores will let users compare city‑to‑city or country‑to‑country momentum on a single scale, revealing emerging AI-hubs sooner and showing whether local policies are translating into thriving AI ecosystems. In short, we will work towards turning raw data into multilayered, geographical insights that complement the broader landscape of rankings and help decision‑makers act with confidence.
Explore the full matrix on our site — and use it to stay firmly in control of the AI landscape of 2025 and beyond.